As part of Best Buy Health, we can help you
bring healthcare home with confidence
With the Current Health enterprise care-at-home platform and the retail power of Best Buy, your organization gets the clinical expertise and wraparound support needed to deliver excellent at-home experiences at scale.
Bring care home for patients
Bring care home for providers.
Bring care home for good.
Bring care home
for patients
Our healthcare system is at a crossroads. We can either go backward into the facility-oriented status quo, or we can redesign healthcare delivery around what is best for patients and consumers.
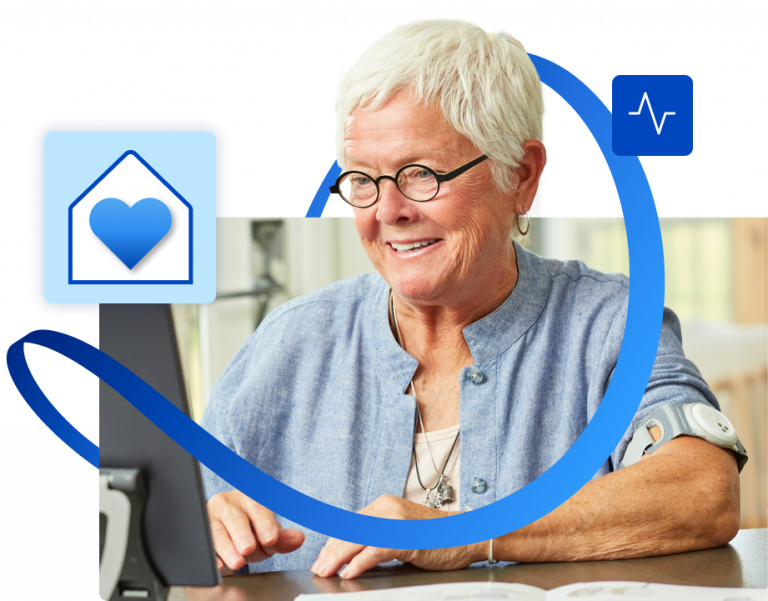
Trusted by leading healthcare organizations
across the industry
Deliver care at home across the continuum
with the simplicity of one platform
Hospital at Home
Transitional Care
Chronic Disease Management
Clinical Trials
Commercial Deployment
Hospital at Home
Expand capacity with Hospital at Home
Extend your hospital into the community when you combine continuous vitals monitoring with 24/7 nursing support to deliver a broad range of acute care at home.
UMass Memorial Health saved more than 6,000 bed days in their first year.
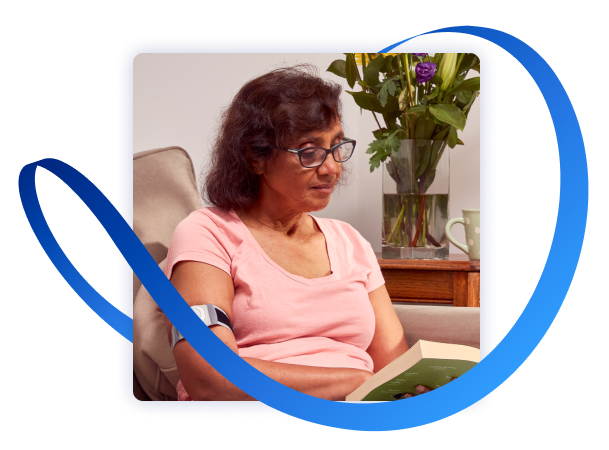